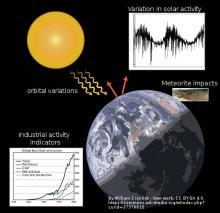
1. Climate change is regarded as a major threat to sustainability
Climate change can be defined as a long-term change in the statistical distribution of weather variables (click here to see examples of weather variables). A related term, "climatic change", was proposed by the World Meteorological Organization (WMO) in 1966 to indicate all forms of climatic variability on long time-scales (more than 10 years), no matter the cause.
During the 1970s, the term climate change replaced climatic change to focus on anthropogenic drivers, as it became clear that human activities had a potential to alter the climate. Today there is a prevailing use of the term "climate change" to mean "anthropogenic climate change". The latter refers to the variation in climate caused by human forces.
Human induced climate variability may be observed at local and global scale. At the global scale, it may be caused by the global increase of greenhouse gases in the atmosphere which in turn may be due to increased human emissions. Greenhouse gases cause the greenhouse effect (see Figure 1) which may be responsible for the recent global warming (see Figure 2 and 3). The primary greenhouse gases in Earth's atmosphere are water vapor, carbon dioxide (CO2), methane, nitrous oxide and ozone.
Figure 1. Greenhouse effect schematic showing energy flows between space, the atmosphere, and Earth's surface. Energy influx and emittance are expressed in watts per square meter (W/m2). By Robert A. Rohde (Dragons flight at English Wikipedia) - This figure was created by Robert A. Rohde from published data and is part of the Global Warming Art project. (https://commons.wikimedia.org/w/index.php?curid=2846779).
Global warming is a major reason of concern today. Figure 2 shows how global temperatures have risen from 1950 to the end of 2013. Current global temperature is measured at a level that is about 1 °C above the pre-industrial era. Even if the difference may look tiny, actually it is an important shift that gives reason for the huge recession of glaciers that was observed in the past 150 years (see Figure 3).
Figure 2. This visualization shows how global temperatures have risen from 1950 through the end of 2013. By NASA (https://www.youtube.com/watch?v=gaJJtS_WDmI en:YouTube, Public Domain, https://commons.wikimedia.org/w/index.php?curid=30879091).
Figure 3. Retreat of the glacier Waggonwaybreen at Magdalenfjord on Svalbard. By Andreas Weith (Own work) [CC BY-SA 4.0 (https://creativecommons.org/licenses/by-sa/4.0)], via Wikimedia Commons.
Figure 4 shows Global mean surface-temperature change from 1880 to 2017, relative to the 1951–1980 mean.
Figure 4. Global mean surface-temperature change from 1880 to 2017, relative to the 1951–1980 mean. The 1951–1980 mean is 14.19 °C (57.54 °F). The black line is the global annual mean, and the red line is the five-year local regression line. The blue uncertainty bars show a 95% confidence interval. By NASA Goddard Institute for Space Studies (http://data.giss.nasa.gov/gistemp/graphs/), Public Domain (https://commons.wikimedia.org/w/index.php?curid=24363898).
In some regions local temperature changes have been larger than global changes. For instance, the anomalies in the European Alps have been about twice those of the global mean. Why, exactly, the Alps should have larger temperature fluctuations has, however, not been explained satisfactorily (Böhm, 2008).
Global warming may be responsible of other changes like rising sea levels, changing precipitation, and expansion of deserts in the subtropics. Increased temperature may also induce additional stress on society related to natural hazards (including earthquakes according to recent assumptions), water resources management, global economy, food production, mobility of people and goods, conservation of buildings and infrastructures, and many others. Moreover, climate change and global warming are perceived as global challenges, which affect the humanity as a whole. Some researchers postulate that climate change may lead the climate system to cross a tipping point. A tipping point is defined as a point when a given system changes from one given stable state to another stable state in a manner that, after the tipping point has been passed, may be irreversible. Thus, climate change may lead to permanent changes in the whole Earth system.
The potentially irreversible impact of climate change captured the attention of the global public and therefore of politicians and policy makers. Several global forums were activated to discuss the problem, like for instance the United Nations Climate Change conference (COP), the World Climate Conference and many others. In 1988, the Intergovernmental Panel on Climate Change was established, which is a intergovernmental body of the United Nations dedicated to providing the world with an objective scientific view of climate change and its political and economic impacts. Meanwhile, enormous resources are continuously dedicated to research on climate change and climate change adaptation.
The striking popularity of the topic and its relevance to international economy and politics originated a global controversy on climate change. The debate is concerned on whether and were global warming is occurring, the potential bias induced by changing measurement techniques, the anthropogenic influence on global warming with respect to natural climate variability, how much and where meteorological variables other than temperature have changed, what will be the future development of climate, whether any action should be taken to mitigate climate change, and if so what that action should be, and so forth. Controversy is further stimulated by predictions of future scenarios that outline an increased risk of severe consequences for humanity. It is well known that people are more concerned by human induced risks - whose potential consequences and evolution cannot not be fully perceived - with respect to natural hazards. Examples of alarming news are given here and here. Also, by looking at the sources of information linked in this page the reader can easily realise differences in opinions and interpretations of climate change.
The current controversy on climate change is detrimental for a scientifically productive assessment of recent climate dynamics and the design of effective strategies for climate change mitigation and adaptation (beware of the difference between climate change mitigation and climate change adaptation, which is not always clear to the public).
The history of science is characterised by several controversies. To provide relevant examples, one may refer to Charles Darwin’s theory of evolution which also caused controversy. Fellow scientists felt Darwin did not have enough evidence to back up his claims. Another example is Galileo, who four hundred years ago was forced by the Catholic Church to deny the heliocentric model of the cosmos – the model in which the planets revolve around the Sun rather than the Sun and planets revolving around Earth. The model had initially been put forward by another famous scientist, Nicolaus Copernicus, in 1543. In 1992, 359 years after his trial, Galileo received a pardon from Pope John Paul II. Wider acceptance of Darwin’s evolutionary theory came more quickly. Fifty years after his theory was published, genetic inheritance was discovered. Subsequent scientific research and observation have provided such a range of evidence that most – but not all – people accept evolution as an explanation for new and changing life forms.
The purpose of this lecture is to provide a brief overview of what natural variability and climate change are, its impact on the water cycle and what are the relevant uncertainties. Knowledge of uncertainty is essential to support engineering design of climate change mitigation and adaptation actions. Neglecting uncertainties in science precludes the possibility to improve understanding and devise efficient ways forward.
Natural climate variability refers to the variation in climate parameters caused by nonhuman forces. Climate varies naturally from one year to the next. Climate can also vary on much longer timescales, from decades to the whole time span of the Earth's life. An interesting, yet technical contribution that brings in light climate variability is Nature's style: Naturally trendy by Tim Cohn and Harry Lins.
We all know about glaciations that are a relevant example of climate variability. Glaciations are long periods of low temperatures, resulting in the presence or expansion of continental and polar ice sheets and alpine glaciers. Earth is currently in the Quaternary glaciation, known in popular terminology as the Ice Age. Figure 5 shows the timing of major glaciations.
Figure 5. Long-term evolution of oxygen isotope ratios during the Phanerozoic eon as measured in fossils, reported by Veizer et al. (1999), and updated online in 2004. Such ratios reflect both the local temperature at the site of deposition and global changes associated with the extent of permanent continental glaciation. CC BY-SA 3.0 (https://commons.wikimedia.org/w/index.php?curid=184098).
The blue line in Figure 5 is a short-term average that was constructed by applying a weighted moving average over a period of 3 Myr to the original 16,692 reported measurements. The gray bar is the associated 95% statistical uncertainty in the moving average. The black line is the long-term average computed over a period of 15 Myr. Both blue and black lines do not show the recent increase of temperature as the moving averages do not allow to see changes in the short time scale. To better inspect the progress in the more recent past Figure 6 provides a graph of reconstructed temperature (blue), CO2 (green), and dust (red) from the Vostok Station ice core for the past 420,000 years. It is interesting to note the dependence of CO2 concentration on temperature. Moreover, we can see inside glaciations the presence of long term cycles, with so-called "interglacial periods" alternating with "glacial periods".
Figure 6. Graph of reconstructed temperature (blue), CO2 (green), and dust (red) from the Vostok Station ice core for the past 420,000 years. By Vostok-ice-core-petit.png: NOAAderivative work: Autopilot (talk) - Vostok-ice-core-petit.png, CC BY-SA 3.0, https://commons.wikimedia.org/w/index.php?curid=10684392.
The causes of ice ages are not fully understood for either the long scale ice age periods or the shorter glacial–interglacial periods within an ice age. It is deemed that several factors are important: atmospheric composition, such as the concentrations of greenhouse gases; changes in the earth's orbit around the Sun known as Milankovitch cycles; the motion of tectonic plates resulting in changes in the relative location and amount of continental and oceanic crust on the earth's surface, which affect wind and ocean currents; variations in solar output; the orbital dynamics of the Earth–Moon system; the impact of relatively large meteorites and volcanism including eruptions of supervolcanoes. A brief review of possible causes is given here.
Changes of climate along shorter time scales are reported by remains, oral traditions and climate proxies like ice cores, tree rings, sub-fossil pollen, boreholes, corals, lake and ocean sediments. A relevant example is the medieval warm period, also known as the Medieval Climate Optimum, or Medieval Climatic Anomaly. It was a time of warm climate in the North Atlantic region that may have been related to other warming events in other regions during that time (See Figure 7). Indeed, there are several oral traditions reporting the presence of woods in the Alps at a much higher altitude than today. Another relevant example is the legend of the lost valley in the Monte Rosa, in Italy. During the "climate optimum" populations of the alpine regions could easily migrate by crossing the alpine passes. After some decades the advance of glaciers made the trip impossible and therefore a legend originated saying that there was a green and peaceful valley that was lost within high altitude ice.
Figure 7. Northern hemisphere temperature reconstructions for the past 2,000 years. CC BY-SA 3.0 (https://commons.wikimedia.org/w/index.php?curid=466264).
The medieval warm period was followed by the Little Ice Age, a period of cooling that occurred from the 16th to the 19th centuries. During that time bad whether was dominant, glacier advanced and caused several problems to agriculture and human settlements. The Little Ice Age ended in the second half of the 19th century or early in the 20th century and since then glaciers are retreating.
Signs of periodical variation on shorter time scales can be found in almost all long measurements (time series) of hydrometeorological variables. The longest rainfall record in the world is the one observed in Padua. A graph of the progress in time of annual rainfall amount and number of wet days can be seen here. Another relevant example is given by Figure 8, showing a 3-D representation of the Po River daily discharge time series (1920-2009). Several local cycles of high and low flows can be identified in the picture. These cycles are well known to hydrologists, as they imply that a reservoir of bigger size is needed if one aims to regulate the river flow, namely, to compensate low flow periods by releasing water storage that was accumulated during high flows. The presence of long term cycles is termed in hydrology Hurst Effect or Hurst-Kolmogorov Effect, according to the terminology introduced by Demetris Koutsoyiannis.
Another significant example of local cycle on temperature records is given by the so-called global cooling, which was a conjecture during the 1970s of imminent cooling of the Earth's surface and atmosphere culminating in a period of extensive glaciation. There is now general agreement that aerosol effects rather than natural variability were the dominant cause of the cooling.
Figure 8. 3D representation of the Po River daily discharge time series (1920-2009). The resulting surface was smoothed by applying a moving average and a Gaussian filter. From A. Montanari, Hydrology of the Po River: looking for changing patterns in river discharge.
The above examples demonstrate that significant climatic fluctuations were observed in the past which cannot be primarily related to human forcing. Some researchers argued that the current acceleration of the warming is unprecedented, but actually the assessment of the pace of past climate variations is affected by relevant uncertainty. Actually, the causes of natural variability are still not completely understood and therefore particular care should be taken when drawing conclusions on its relationship with anthropogenic forcing and its importance with respect to it.
Human activity has an influence on climate at several spatial scales. At local scale, waste heating in cities and land use change cause the heat island effect, which implies a temperature difference between the inner part of a human settlement and its surroundings. The temperature difference is usually larger at night than during the day, and is larger when winds are weak. Furthermore, deforestation, agricultural activities and irrigation can significantly change evaporation and transpiration, therefore implying a modification of local temperature and rainfall regime.
At the global level, human activity impacts on climate through the emissions of greenhouse gases, which were discussed above. Simulations by climate models show that increasing concentration of CO2 in the atmosphere leads to global warming, at a rate that may bring the global temperature at more than 2 °C above the pre-industrial level by 2100, if CO2 emissions are not reduced. Increase in temperature in turn implies that saturation vapor pressure increases 7% per ̊C of warming, according to the basic physics expressed in the Clausius-Clapeyron equation, relating saturation vapor pressure to temperature. However, the increased saturation vapor pressure does not necessarily imply that more water is available in the atmosphere to generate precipitation. Indeed, feedback effects - that wil be discussed in the following sections - may reduce evaporation therefore conditioning the atmospheric water content.
In general, climate feedbacks play an important role, as they may amplify or annihilate the effect of climate forcings. Thus, they are essential to determine climate sensitivity to human impact and future climate state. Feedback is the process in which changing one quantity changes a second quantity, and the change in the second quantity in turn changes the first. Positive feedback amplifies the change in the first quantity while negative (or balancing) feedback reduces it. Climate feedbacks are inherently complex and even more complex when considered as part of the integrated global climate system. They are not completely understood and some may not have been discovered yet.
Human impact on climate may be assessed through deductive or inductive approaches. Deductive approaches are based on reasoning that leads from premises (assumptions, based on our knowledge) to reach conclusions. They typically infer future behaviours by exploting predictability of system dynamics through mathematical models. Inductive procedures start from data analysis to assess the current state of the system to build knowledge that supports premises (assumptions).
In climate science deductive approaches are largely used by applying climate models. Inductive approaches are typically applied by analysing observed data and relating the detected changes to human intervention. Data analysis is frequently applied by engineers for sizing climate change adaptation stuctures, as they correspond to the usual practice of professional design. In view of their uncertainty climate models cannot be directly used for design, but their output is neverheless useful to depict future scenarios that can be used to study the resilience of the designed solution. The legal responsibility of the engineer implies that uncertainty is properly taken into account and the risk of failure is minimised. This is the reason why engineeers prefer to rely on data to support with facts and evidence the model predictions.
Actually, our incomplete understanding of climate dynamics limits our capability to predict future climate and human impact through a deductive procedure alone. Therefore, it is advisable to make a synthesis of deductive and inductive approaches: inductive approaches allow us to improve our understanding of the current state and its past evolution. They may also allow to make predictions of future states, by extrapolating past behaviours. To the extent that data analysis provides support to the premises (assumptions) of climate models, deductive and inductive approaches can be combined for assessing human impact and performing technical design of mitigation and adaptation strategies. This procedure will be discussed in Section 8.
In Section 4 below we discuss recent changes in hydrometeorological variables basing on data analysis.
Climate change implies variations in meteorological variables which in turn induce changes in hydrological fluxes. Hitherto we discussed how climate change affects temperature. In this section we focus on precipitation and sea level, which are relevant for water resources management. Changes in river flow will be analysed in the following section.
Precipitation is formed by all liquid and solid water particles that fall from clouds and reach the ground. These particles include drizzle, rain, snow, snow pellets, ice crystals, and hail. Precipitation occurs when a portion of the atmosphere becomes saturated with water vapor, so that the water condenses and falls. It is measured in terms of height of water column that accumulates over an horizontal surface during a given observation time step. Figure 9 shows the long-term mean precipitation by month over the globe.
Figure 9. Long-term mean precipitation by month (Karger et al., 2017). By Greenmind1980 - Own work, CC BY-SA 4.0 (https://commons.wikimedia.org/w/index.php?curid=50555801).
Climate change of course may impact precipitation. In fact, the above mentioned increase of saturation vapor pressure after global warming implies that more water may be available in the atmosphere to produce rainfall. However, mean evaporation and precipitation do not necessarily increase at the above rate, because of the atmospheric energy budget constraint. Accordingly, precipitation may only increase at the same rate as the atmosphere can release energy by radiation, which is only 1-2%/°C. Moreover, rainfall also depends on the circulation of winds. If circulation slows down, rainfall does not increase.
Once again, it is important to take into account that climate dynamics is still poorly known. It was already mentioned that the Earth system compensates for perturbations by activating feedbacks that are not completely understood. A relevant example is given by the so-called evaporation paradox. While it is expected that evaporation increases under global warming, actually observations of pan evaporation across the globe show a declining trend (see, for instance, Roderick and Farquhar, 2002).
Two possible explanation have been put forward for the decline in pan evaporation.
- The first explanation is that pan evaporation has decreased because evaporation from the environment surrounding the pan has increased. Accordingly, the vapor pressure deficit should have decreased. However, data from the United States show that its average has remained virtually constant over the past 50 years.
- The second explanation invokes reductions in solar irradiance resulting from more clouds and/or aerosols and is generally consistent with the independent suggestion that increased pollution would weaken the pace of the hydrological cycle.
Like for any other climatic variable, precipitation changes may be assessed by data analysis (inductive approach) and/or climate model simulations (deductive approach). Data analysis is criticized for the limited global coverage of local data, lack of data over the oceans and observational uncertainty, which is also due to changes in monitoring methods. On the other hand, climate models are criticized for their limited reliability in terms of precipitation prediction at local level and short time scale.
The results of several studies depict heterogeneous precipitation changes that also depend on the time scale. Hydrological variables like rainfall and river flow are more difficult to measure and predict than temperature. In fact, rainfall and river flows are highly non-linear, with an asymmetric probability distribution, while temperatures are closer to linearity and Gaussianity. Moreover, changing climatic conditions have different effects on weather and hydrology, depending on local features like orography, geomorphology and soils type in the catchment. Dependence on local conditions is a distinguishing feature of hydrology that can make the effect of climate change less predictable and diversified.
Data show that precipitation amount has generally increased over land north of 30°N from 1900 to 2005 but has declined over the tropics since the 1970s. Globally there has been no statistically significant overall trend in precipitation over the past century, although trends have varied widely by region and over time. It seems that eastern portions of North and South America, northern Europe, and northern and central Asia have become wetter. The Sahel, the Mediterranean, Southern Africa and parts of Southern Asia have become drier. Over the contiguous United States, total annual precipitation increased at an average rate of 6.1% per century since 1900, with the greatest increases within the East North Central climate region (11.6% per century) and the South (11.1%). Hawaii was the only region to show a decrease (−9.25%) (data from this Wikipedia page).
In terms of extreme events, results show again a marked heterogeneity. Several studies show that there has been an increase in the number of heavy precipitation events over many areas during the past century, as well as a potential increase since the 1970s in the severity of droughts—especially in the tropics and subtropics. Papalexiou and Montanari (2019) analyzed long rainfall intensity records over the globe and found that the annual number of the largest 30 values in a 30-year long time window is weakly increasing along time at the global level. The increasing tendency is determined by the pattern of the Northern hemisphere, while no significant increase is observed in the Southern hemisphere. Figure 10 and 11 provide a synthesis of the above results.
Figure 10. Mean trend values in 5° × 5° grid cells in extreme daily precipitation over the period 1964–2013. Maps show trends in (a) frequency as number of extreme events per decade and (b) magnitude as mm per decade. From Papalexiou and Montanari (2019).
Figure 11. Mean trend values of frequency (events/decade) of extreme daily precipitation in large geographical zones over the period 1964–2013. Maps show the results for globe, North hemisphere Northwest
quadrant, Northeast quadrant, and Southeast quadrant (zones are indicated by insets with global maps). The smooth line shows the seven‐year moving average. From Papalexiou and Montanari (2019).
In terms of precipitation intensity of extreme events, results are again heterogeneous. Rainfall extremes appear to be changing around the world but there is little information on how extreme short-duration events might change. Some studies suggest that hourly rainfall magnitude might increase beyond thermodynamic expectations with global warming; that is, above the Clausius–Clapeyron (CC) rate of ~6.5% °C−1. However, there is limited evidence of such increases in long-term observations. Guerreiro et al. (2018) assessed continental-average changes in the magnitude and frequency of extreme hourly and daily rainfall observations from Australia over the years 1990–2013 and 1966–1989. They found that "increases in daily rainfall extremes are consistent with CC scaling, but are within the range of natural variability". And: "In contrast, changes in the magnitude of hourly rainfall extremes are close to or exceed double the expected CC scaling, and are above the range of natural variability, exceeding CC × 3 in the tropical region (north of 23° S)". The CC x 3 scaling is the highest value so far detected through data analysis. Other assessments have been carried through climate models, whose uncertainty is significant (it was discussed above). Papalexiou and Montanari (2019) analyzed daily records with coverage extended to the whole world. Their results are characterized by marked heterogeneity. Increasing trends seem to prevail in daily rainfall but their magnitude is limited.
One should take into account that trend analysis is in turn affected by significant uncertainty. It is usually carried out by estimating a linear trend over a given time series. Linear trend estimation expresses data as a linear function of time. The linear assumption is introduced to increase robustness therefore reducing uncertainty. Nevertheless, even when dealing with long time series uncertainty remains relevant, which in turn implies that the trend pattern along time is not clear.
To summarize, several studies pointed out an increase in frequency and intensity of extreme precipitation in recent decades, while average values seem less impacted. The order of magnitude of the increase in frequency of extreme events has been reported to be about 5% at the global level in the last 40 years (Papalexiou and Montanari, 2019), while the highest detected increase of intensity has been found in Australia and is about 18% per °C (namely, about 18% with respect to the pre-industrial level). The above increases maybe related to global warming.
An excellent review of studies on sea level rise and its causes is provided by Curry (2018), which is the source of much of the content of this section.
Sea level changed significantly during the history of Earth. Figure 12 and 13 show changes in sea level since 24 thousands of years ago. Since the end of the little ice age, around 1880, the average global sea level has been steadily rising. Between 1900 and 2016 sea level rose by approximately 16 to 21 cm. Data from satellite radar measurements show an accelerating rise of 7.5 cm from 1993 to 2017, which is coherent with the pace of global warming. The rise is due to thermal expansion of seawater - which is responsible for about half of the rise - and melting of ice sheets and glaciers. Sea level rise is not uniformly distributed on Earth, as it is influenced by local factors including tectonic effects, subsidence, currents and storms.
Figure 12. Changes in sea level since the end of the last glacial episode. This figure was prepared by Robert A. Rohde from published data, and is incorporated into the Global Warming Art project. CC BY-SA 3.0 (https://commons.wikimedia.org/w/index.php?curid=479979).
Figure 13. Changes in sea level during the last 25 thousands of years. By Vivien Gornitz - The Great Ice Meltdown and Rising Seas: Lessons for Tomorrow, NASA News, June 2012, Public Domain (https://commons.wikimedia.org/w/index.php?curid=42012722).
Like for several other impacts of climate change, a widespread alarmism on sea level rise developed during the past decades. Concerns are not about the past sea level rise, but rather focused on the projections for the 21st century, the more pessimistic ones predicting a rise of several meters. Figure 14 shows a map prepared by NASA highlighting in red the land that would be flooded if sea level rose 6 meters with respect to current level.
Figure 14. Map of the Earth with a six-meter sea level rise represented in red. By NASA (https://www.flickr.com/photos/11304375@N07/6863515730/). Additional source (http://www.livescience.com/19212-sea-level-rise-ancient-future.html) Live Science. Public Domain (https://commons.wikimedia.org/w/index.php?curid=40213299).
Given that we know that sea level changed significantly in the past, a relevant question is whether the current trend is unprecedented or not. A comprehensive review of studies on past sea level is given by Curry (2018), who points out that Hubert Lamb’s (1995) book Climate History and Modern World offers the following note:
“The water level may have dropped by 2 meters [6.5 feet] or more between 2000 and 500 BC. What does seem certain is that there was a tendency for world sea level to rise progressively during the time of the Roman Empire, finally reaching a high stand around 400 AD comparable with, or slightly above, present”.
A relevant question is whether the sea level trend observed during the XXth century is unprecedented. It is not easy to answer to this question since we do not have long term observations and the available records are very sparse.
Time series resulting from different global sea level rise assessments since 1880 are shown in Figure 15. Differences in the data are due to tide gauge location, vertical land motion that may be caused by subsidence and tectonic activity and statistical analysis methods.
Figure 15. Global mean sea level reconstructions over the period 1880-2012. Black line shows the three-year moving average. Red line shows data derived from satellite measurements. Data derived from 23 different gauges. By Robert A. Rohde, modified by A. Montanari - Image:Recent Sea Level Rise.png by Robert A. Rohde. This figure was prepared by Robert A. Rohde from published data, and is incorporated into the Global Warming Art project. Translation in french and conversion to svg was done by SuperManu, CC BY-SA 3.0 (https://commons.wikimedia.org/w/index.php?curid=3003623).
Figure 16 shows recent sea level rise since 1992.
Figure 16. Sea Level Rise as measured by the TOPEX/Poseidon and Jason-1 satellites. By http://earthobservatory.nasa.gov/IOTD/view.php?id=6638, Public Domain (https://commons.wikimedia.org/w/index.php?curid=8016880).
The fifth assessment report (AR5) IPCC concluded that:
“It is very likely that the mean rate of global averaged sea level rise was 1.7 [1.5 to 1.9] mm/year between 1901 and 2010 . . . and 3.2 [2.8 to 3.6] mm/year between 1993 and 2010. It is likely that similarly high rates occurred between 1920 and 1950.”
The above conclusion proves that cyclical behaviors have been observed in sea level, which are not directly related to the rate of global warming.
Satellite measurements of global sea level have been available since 1992, which has improved our understanding of the variability of global sea level rise. The average rate of sea level rise during this period is estimated in the range 2.6 to 3.2 mm/year (Horton et al, 2018). Temporary cycles have been observed which may be associated to the El Niño Southern Oscillation (ENSO) and La Niña (2011). These variations are associated with changes in the global hydrological cycle and the distribution of rainfall over land versus over ocean. There is a tendency for land water deficit including groundwater (and temporary increase of the global mean sea level) during El Niño events and the opposite during La Niña (Fasullo et al. 2013).
As concluded by Munk (2007) with respect to the satellite measurements:
“At best, the determination and attribution of global-mean sea-level change lies at the very edge of knowledge and technology. Both systematic and random errors are of concern, the former particularly, because of the changes in technology and sampling methods over the many decades, the latter from the very great spatial and temporal variability. It remains possible that the database is insufficient to compute mean sea-level trends with the accuracy necessary to discuss the impact of global warming – as disappointing as this conclusion may be.”
The IPCC AR5 acknowledges that a long time series is needed to detect acceleration in sea level rise from human-caused climate change:
“While technically correct that these multidecadal changes represent acceleration/ deceleration of sea level, they should not be interpreted as change in the longer-term rate of sea level rise, as a time series longer than the variability is required to detect those trends.” [IPCC AR5 WGI Section 3.7.4]
Both gauges data and satellite observations concur in reporting a sharp increase of the sea level rise trend after 1990. Besides the above mentioned similarity of the post-1990 trend with the 1920-1950 one, it is interesting to note that the Northern Hemisphere oceans play a small role in the post-1990 acceleration, which is mainly determined by hot spots located in the Southern Hemisphere. Figure 17 shows a global map of sea level rise from 1992 to 2013.
Figure 17. Global map of sea level rise from 1992 to 2013. By Giorgiogp2 - Own work, CC BY-SA 3.0 (https://commons.wikimedia.org/w/index.php?curid=9400992).
The detection of human induced signals in sea level rise is made more complicated by the existence of a 60-year signal cycle in sea level that appears in every ocean basin (Chambers et al. 2012). The presence of such cycle is consistent with high rates of sea level rise observed during the 1940’s and 1950’s. The presence of periodicity was recognized by the IPCC AR5:
“A long time-scale is needed because significant multidecadal variability appears in numerous tide gauge records during the 20th century. The multidecadal variability is marked by an increasing trend starting in 1910–1920, a downward trend (i.e., leveling of sea level if a long-term trend is not removed) starting around 1950, and an increasing trend starting around 1980.” [IPCC AR5 WGI Chapter 3]
In conclusion, basing on the available knowledge and in consideration of the related uncertainties, there is weak support to conclude that the recent rate of sea level rise, that is, roughly 3 mm/yr, is unusual and human induced
How much the sea level is expected to increase in the 21st century? This is another relevant question for planning urban development and water resources management. Predictions of sea level rise were computed by the IPCC basing on the output from climate models for given future emissions scenarios. Figure 18 summarizes the IPCC AR5 global temperature and sea level rise projections for 2046-2065 and 2081-2100. The upper limit of the likely range of projected sea level rise for 2081-2100 for the extreme RCP8.5 emission scenario is 0.82 m.
Figure 18. IPCC AR5 global temperature and sea level rise projections for 2046-2065 and 2081-2100 [IPCC AR5 WG I Summary for Policy Makers Table SPM.2].
For comparison, let us note that if the current trend of 3 mm/yr continues up to the end of the century, the sea level would rise of about 25 cm. These estimates of sea level rise are much lower with respect to more pessimistic predictions sometimes proposed by the media, who mention potential increases of sea level of several meters with respect to the current state. These predictions assume that abrupt changes in climate may occur that may induce a rapid melting of ice sheet. Let us consider that predicting whether or not elements of Earth's system will abruptly tip into a different state is extremely challenging. The above mentioned limited extension of historical climate does not allow us to test the skill of computer models used to predict future environmental change.
Climate change affects the water cycle, by inducing relevant societal implications. Changes in river flow and groundwater dynamics impact on water resources management, natural hazards like floods and droughts as well as energy and food production through the water-food-energy nexus. In Spring 2017 a research focusing on Arctic river ice revealed what is believed to be “the first case of large-scale river reorganization as a result of human-caused climate change,” according to the Washington Post. In 2018, an assessment by the Joint Research Center (JRC) of the future water resources of the Danube River Basin finds that climate change beyond 2 °C would lead to increased flooding and water scarcity, significantly drier summer months and substantial flood damage in cities along the Danube River and its tributaries.
The above predictions are mainly based on model simulations (deductive approach). Indeed, several hydrological studies were recently proposed by the literature in the attempt to decipher the impact of climate change on river flow. These studies are carried out by feeding a hydrological model with projections given by climate models. A cascade of models is applied, with uncertainties progressively accumulating (Bloeschl and Montanari, 2010). In fact, even if catchment modelling is affected by less uncertainty than climate modelling, uncertainty of hydrological simulations and predictions is still relevant. There are limits to model accuracy at the catchment scale, which are more stringent than what is usually perceived. Some processes, like groundwater flow, can hardly be observed and the control volume of models is characterized by a complex geometry than cannot be described in detail. Field experiments on infiltration, groundwater recharge and so forth regularly give different outcomes even when all the input variables and constraints that are considered by the more complex models are identical. The reason is the presence of inherent uncertainty which will never be removed (Montanari and Koutsoyiannis, 2012). Uncertainty is due to imprecise description of the geometry, uncertainty in model inputs and boundary conditions, presence of chaotic behaviors, which imply a marked sensitivity of model outputs to initial conditions, and so forth. When hydrological uncertainty is superimposed to climate uncertainty the results may be not representative of future conditions in a deterministic sense.
Another way of assessing changes in river flow is the analysis of long term observations. These typically show a different picture. It appears from data that the mean value of river flow is not significantly changed but in very specific situations where human impact is massive and exerted through direct intervention on river network and catchment. When looking at extreme values - floods and droughts - a distinctive pattern does not emerge as well. In some regions floods and droughts look exacerbated, but in other locations one does not see remarkable differences.
We may consider the Po River - the longest river in Italy - as a significant example. In fact, media repeatedly mentioned the Po River as a typical example where climate change increased water scarcity and flood risk (see, for example, here).
The Po River is intensively monitored. Observations of daily river flows are available at several Po River cross sections from the early 20th century. Figure 7 above shows a 3-D plot of daily river flows at Pontelagoscuro - near Ferrara, where the closure section of the Po River is conventionally located - from Jan 1st, 1920 to Dec 31st, 2009. The figure highlights a period of high flows during the 90s of the XXth century. The occurrence of two major floods in 1994 and 2000, whose return period was at that time considered to be above 50 years, induced the concern that climate change was increasing flood risk. After 2000 no significant floods occurred along the Po river. Figure 19 shows a picture taken in the Parma district at the time when the hydrograph of the 2000 flood peaked.
Figure 19. November 2000, flood of the Po River (Italy). The picture was taken in Parma (Ph. Alberto Montanari).
Figure 20 shows a plot of annual maxima of daily average flow, along with the results of linear trend estimation. The latter is carried out over a moving window of 50 years, to show how the pattern has changed over the observation period.
Figure 20. Annual maxima of the Po River at Pontelagoscuro daily discharge series (1920–2009). Linear regression is estimated over a moving window of 50 years. From A. Montanari, Hydrology of the Po River: looking for changing patterns in river discharge.
By looking at the progress of the peak flows a clear pattern cannot be distinguished. The regression lines shows that there is significant uncertainty when trying to interpolate the data with a linear function, even if the length of the regression period is quite extended (50 yrs).
A large scale study of recent changes in flood peaks in Europe was performed by Hall et al. (2014, open access paper) and turned out to be highly cited. The authors state that:
"There is growing concern that flooding is becoming more frequent and severe in Europe. A better understanding of flood regime changes and their drivers is therefore needed."
It is recalled in the above study that several reasons may contribute to flood regime changes, including river training, construction of dikes, groynes and weirs, operation of artificial reservoirs, urbanisation, agricultural management practices, flood retention basins, natural climate variability and climate change. Figure 21 shows a schematic summarising the observed flood changes in Europe derived from a review of previous studies using different not directly comparable change analysis methods and time periods. It can be seen that the reported patterns are indeed heterogeneous.
Figure 21. Schematic summarising the observed flood changes in Europe derived from studies reviewed by Hall et al. (2014). Detection was carried out by using different and not directly comparable change analysis methods and time periods. Arrows in the schematic indicate the majority of trends including regions with weak and/or mixed change patterns. Areas with no/inconclusive studies due to insufficient data (e.g. Italy) and inconclusive change signal (e.g. Sweden) are not shown. From Hall et al. (2014).
Hall et al. (2014) basing on the above review note that:
"Overall, it is difficult to issue a general statement on flood changes in Europe due to the diversity of processes, different observation periods, and the variety of methods applied."
A recent study by Bloeschl et al. (2019) pointed out that floods magnitude is increasing in some regions of Europe and decreasing in others. In particular floods seems to be decreasing in the mediterranean area. Their study refers to medium to large size basins and therefore may not take into account changes in flash floods, which tipically occur in small basins and are therefore triggered by short time storms.
A previous study by Bloeschl et al. (2017) reported significant changes in seasonality of floods in Europe, due to global warming. Other recent studies under development note that changes in flood regime in Europe may be detected where snowmelt and glacier melting plays an important role in the formation of flood flows. The picture that emerges seems to point out that flood changes are not primarily due to changes in rainfall, but rather to changes in snowmelt dynamics.
When the focus turns on flash floods, that are typically occurring in small and highly urbanised catchments, the changes seems to be mainly due to land use change and river training, rather than climate change.
There is the general feeling that climate change has increased the drought risk. Recent examples of droughts that look unprecedented are the drying of California and the Millenium Drought in Australia. NOAA reported that the Californian drought may be linked to the intensity of El Niño Southern Oscillation (ENSO), and that the record-high temperature that accompanied the recent drought may have been amplified due to human-induced global warming. El Niño Southern Oscillation (ENSO) has been found to be intimately related to the Millenium drought as well. These droughts manifested themselves as a long sequence of dry years. This event is called a "multi-year" drought and represent a major hazard for modern society. It is interesting to wonder whether the frequency of multi-year droughts is increased after global warming. Aswering such challenging question requires some more information on El Niño.
El Niño is a naturally occurring weather pattern. It is the warm phase of the El Niño–Southern Oscillation (ENSO) which in turn is an irregular periodic variation in winds and sea surface temperatures over the tropical eastern Pacific Ocean, affecting climate of much of the tropics and subtropics. El Niño occurs when ocean temperatures in the Pacific Ocean near the equator are warmer than usual. The cooling phase is instead called La Niña. The Southern Oscillation is the accompanying atmospheric component: El Niño is accompanied by high air surface pressure in the tropical western Pacific and La Niña with low air surface pressure there. Figure 22 shows a pictogram of the above penhomena during La Niña phase, along with the corresponding position of the Pacific termocline and the Walker wind circulation which is a driver of the ENSO.
Figure 22. Diagram of La Niña phase of the Southern Oscillation. The Walker circulation is seen at the surface as easterly trade winds which move water and air warmed by the sun towards the west. The western side of the equatorial Pacific is characterized by warm, wet low pressure weather as the collected moisture is dumped in the form of typhoons and thunderstorms. The ocean is some 60 centimetres (24 in) higher in the western Pacific as the result of this motion. The water and air are returned to the east. Both are now much cooler, and the air is much drier. An El Niño episode is characterised by a breakdown of this water and air cycle, resulting in relatively warm water and moist air in the eastern Pacific.
El Niño typically occurs every two to seven years. The 2015-2016 El Niño was called a “super” El Niño, the worst in 15 years. The two previous super El Niños occurred in 1982-1983 and 1997-1998.
In normal conditions strong trade winds blow from east to west across the Pacific Ocean around the equator. The winds push warm surface ocean water from South America west towards Asia and Australia, and cold water comes up from the deep ocean in the east to take its place along South America. The temperature disparity across the Pacific keeps the trade winds blowing, namely, the temperature difference and the trade winds reinforce each other. The accumulation of warm water in the west adds heat to the air creating unstable weather, which is why the western Pacific region is warm and rainy, while the eastern Pacific is cooler and drier. In an El Niño year, the trade winds weaken or break down. The warm water that is normally pushed towards the western Pacific washes back across, piling up on the east side of the Pacific from California to Chile. The result is a rainy weather in the west of the America and a dry period in the Eastern Pacific. El Niño can affect weather around the world by influencing high and low pressure systems, winds and precipitation.
Given the above mentioned intimate relationship between ENSO and multi-year droughts, it is interesting to investigate whether there is a relationship between El Niño and global warming. It is not an easy question to answer as climate models do not give a coherent scenario for the future frequency of El Niño events. Some models suggest the ENSO cycle will become more intense, others predict that it will weaken, and some find there will be little change. Vecchi and Witterberg note that:
"....the sensitivity of the response of ENSO to the character of ENSO in these [GCM] models may suggest a way to extrapolate the model results to that of the real climatesystem"
and
"....our understandingof the basic physics of ENSO in these models must improve before confidence can be placed on such extrapolation".
There is a very large variation in ENSO statistics (frequency/magnitude) over time, and so detecting a shift due to climate change is very challenging. Also, we do not have a long time series of ENSO available to investigate its natural variability.
The Italian context is interesting as we have long time series of rainfall and river flow that show the repeated occurrence of multi-year droughts in the past. Figure 23 shows the progress of annual rainfall in Bologna from 1813 to 2019. Multi-year droughts are highlighted in gray. Figure 24 show the progress of summer rainfall (June-July-August). It can be seen that the frequency of multi-year droughts in apparently decreased in the recent past.
Figure 23. Annual rainfall in Bologna with multi-year droughts in grey.
Figure 24. Annual summer rainfall (June-July-August) in Bologna with multi-year droughts in grey.
Another interesting example is again the Po River in Italy. Figure 25 shows a plot of annual minima of daily average flow, along with the results of linear trend estimation. The latter is carried out over a moving window of 50 years, to show how the pattern has changed over the observation period.
Figure 25. Annual minima of the Po River at Pontelagoscuro daily discharge series (1920–2009). Linear regression is estimated over a moving window of 50 years. From A. Montanari, Hydrology of the Po River: looking for changing patterns in river discharge.
The figure highlights a decrease of flows during the summer season after the year 2000. A similar patter was observed during the 40s of the XXth century. The latter drought was particularly severe. By looking at the past history of average annual precipitation over Italy, one again notes the presence of cyclical patterns that may be related to the above mentioned Hurst-Kolmogorov Effect. During the XIXth century such cycles had a period of about 30 years. During the XXth century the period reduced to about 10 years. The period 1800-1860 was rainy and cold. After 1860 we did not observe significant changes in mean annual precipitation. During the XXth century we observed the above mentioned dry period during the 40s, a rainy period between 1950 and 1980 and a slight decrease of precipitation after 1995 until today. The driest year since the beginning of the XIXth century was 1945, with -30% with respect to the 1961-1990 average. The wettest year was 1826, with +41%.
As for floods, it is difficult to be conclusive on the attribution of recent droughts to climate change. Figure 25 shows no clear tendency and droughts are affected by several factors, including natural variability. There is high uncertainty on the impact on drought frequency of each potential drought cause. Some sources attribute to climate change the observed increase of drought length, which would imply an increased periodicity of precipitation patterns. Again, this effect may be due to natural variability as well.
One may conclude by referring once again to Tim Cohn and Harry Lins' Nature's style: Naturally trendy, where they conclude:
"For example, with respect to temperature data there is overwhelming evidence that the planet has warmed during the past century. But could this warming be due to natural dynamics? Given what we know about the complexity, long-term persistence, and non-linearity of the climate system, it seems the answer might be yes. Finally, that reported trends are real yet insignificant indicates a worrisome possibility: natural climatic excursions may be much larger than we imagine. So large, perhaps, that they render insignificant the changes, human-induced or otherwise, observed during the past century."
6. Climate change as predicted by climate models
The most used approach to assess human impact on climate is based on the use of climate models. It is a deductive method that is based on our current knowledge and underlying assumptions, as any model is. Climate models are not meant to reproduce past climate - although they can be adapted to this latter purpose - and are not conceived to provide a deterministic prediction of what will happen in the future. The aim of climate models is to produce future scenarios that may occur on the basis of our understanding of the climate system and given assumptions on future scenarios of CO2 emissions. Climate models are thus not meant to reproduce variables that have been - or could be - observed, but rather to simulate what we could expect in the future if models and assumptions are true. As such, uncertainty for climate simulations cannot be rigorously estimated, as a formally correct approach to uncertainty assessment requires the comparison with what has been observed. By producing an ensemble of simulations with different working hypotheses, boundary conditions and so forth one can test the sensitivity of model output to the underlying assumptions. The resulting simulation envelope, however, only gives an idea of the difference among models and is not a formal estimate of uncertainty. If all models are wrong, the simulation envelop may not contain the true values that will occur. An interesting note in this respect if offered by Brown et al. (2012):
A problem with this approach is that GCM projections are relatively poor scenario generators. They describe a “lower bound on the maximum range of uncertainty” (Stainforth et al., 2007). Even a large multimodel ensemble provides relatively few samples in a typical analysis relative to the size possible through stochastically generated risk analysis. Larger ensembles are becoming available, such as via the climate prediction.net experiment (Stainforth et al., 2004) but still face issues of biases that may preclude the discovery of plausible climate risks.
Figure 23 shows simulations by several climate models under an assigned future emission scenario (SRES-A2).
Figure 23. Climate model predictions for global warming under a given (SRES-A2) emissions scenario relative to global average temperatures in 2000.
Koutsoyiannis et al. (2008) compared the output of various models to temperature and precipitation observations from eight stations with long (over 100 years) records from around the globe. The results showed that models performed poorly, even at a climatic (30-year) scale. They also found that the claim that models can perform better at larger spatial scales is unsupported. Padrón et al. (2018) noted that projections of future water availability vary substantially across different models and found that such disagreement can be reduced by placing more confidence on models that more accurately reproduce observed rainfall amounts. In particular, they showed that previous projections of very extreme future changes in water availability are less likely to occur. Moon et al. (2018) investigated how well current generation models represent drought persistence over the twentieth century and the recent past. They highlighted that the considered models tend to underestimate drought persistence at monthly and annual time scales. Joseph et al. (2018) compared the contributions of uncertainty from hydrological parameterization in the hydrological projections of climate change to that generated from the use of multiple climate models. The Ganga River Basin in India was selected as the study region. They conclude that
"from hydrological point of view, the multi-model climate change projections collectively do not provide any confidence to use them for adaptation".
The overall conclusion of the above discussion is that particular care should be taken when assessing human impact on climate through climate models, especially if the output is expected to support engineering design, for which a reliable uncertainty assessment is an essential requirement. Actually, uncertainty range of climate models is larger than the detected human impact in several cases, especially when dealing with variables other than temperature.
Nevertheless, what-if scenarios produced by climate model are useful to provide projections of future climate statistics, provided their uncertainty is duly taken into account. This issue will be further discussed in Section 9.
Climate change mitigation consists of actions to limit the magnitude or rate of climate change. Actions primarily consists of reductions in human emissions of greenhouse gases. Mitigation may also be achieved by increasing the capacity of carbon sinks, e.g., through reforestation or other methods.
Emission reductions have been discussed in several international conferences like the United Nations Framework Convention on Climate Change, which issued the Kyoto Protocol and the United Nations Climate Change conference (COP). The target is to reach international agreements that bind countries to emission reduction targets. In 2010, Parties to the UNFCCC agreed that future global warming should be limited to below 2.0 °C relative to the pre-industrial level. With the Paris Agreement of 2015 this was confirmed, but was revised with a new target laying down "parties will do the best" to achieve warming below 1.5 °C. Current level of warming is recognized around 0.8 °C.
Emission reduction has several impacts on economy and politics and therefore reaching an international consensus is not an easy task. The problem is also complicated by the uncertainty related to the effectiveness of reducing emissions. While the target certainly implies relevant benefits, including reduction of air pollution, it would not lead to significant mitigation of climate change if the latter was mainly due to natural variability. Given the high societal cost associated to emission reduction, the above question is highly relevant to sustainable development.
Increasing carbon sinks is also a potentially relevant solution. Examples are direct air capture, and enhanced weathering technologies such as storing it in geologic formations underground. These processes are sometimes considered as variations of sinks or mitigation, and sometimes as geoengineering (Figure 24).
Figure 24. Schematic showing both terrestrial and geological sequestration of carbon dioxide emissions from a coal-fired plant. By LeJean Hardin and Jamie Paynederivative work: Jarl Arntzen (talk). CC BY-SA 3.0 (https://commons.wikimedia.org/w/index.php?curid=7268088).
The cost of carbon sequestration is uncertain, as well as its effectiveness, which may be limited by leakage of stored CO2. The process also requires a significant amount of energy.
Climate change adaptation is a response to climate change aiming at reducing the vulnerability of social and biological systems to relatively sudden change and thus offset the effects of global warming. While climate change mitigation is a typical top-down approach, climate change adaptation is an example of the so-called "bottom-up" approach to sustainability, which is discussed here. Climate change adaptation focuses on resilience and sustainability of current societal systems and therefore it is based on "people" rather than politics. Also, climate change adaptation involves actions to be taken at several spatial scales and therefore distributes the responsibility for social security over local, regional and country administrations. Adaptation is especially important in developing countries since those countries have a reduced capacity and potential for humans to adapt (called adaptive capacity).
Scheraga and Grambsch (1998) identify 9 fundamental principles to be considered when designing adaptation policy:
- The effects of climate change vary by region;
- The effects of climate change may vary across demographic groups;
- Climate change poses both risks and opportunities;
- The effects of climate change must be considered in the context of multiple stressors and factors, which may be as important to the design of adaptive responses as the sensitivity of the change;
- Adaptation comes at a cost;
- Adaptive responses vary in effectiveness, as demonstrated by current efforts to cope with climate variability;
- The systemic nature of climate impacts complicates the development of adaptation policy;
- Maladaptation can result in negative effects that are as serious as the climate-induced effects that are being avoided;
- Many opportunities for adaptation make sense whether or not the effects of climate change are realized.
While the above principles are informative from a policy maker point of view, they do not provide technical guidance for the design of climate adaptation strategies. A list of possible interventions to climate change adaptation is given here and reads as follows:
- Installing protective and/or resilient technologies and materials in properties that are prone to flooding;
- Changing to heat tolerant tree varieties;
- Rainwater storage to deal with more frequent flooding rainfall – Changing to water permeable pavements, adding water-buffering vegetation, adding underground storage tanks, subsidizing household rain barrels;
- Reducing paved areas to deal with rainwater and heat;
- Adding green roofs to deal with rainwater and heat;
- Requiring waterfront properties to have higher foundations;
- Raising pumps at wastewater treatment plants;
- Surveying local vulnerabilities, raising public awareness, and making climate change-specific planning tools like future flood maps;
- Incentivizing lighter-colored roofs to reduce the heat island effect;
- Installing devices to prevent seawater from backflowing into storm drains;
- Installing better flood defenses, such as sea walls and increased pumping capacity;
- Buying out homeowners in flood-prone areas;
- Raising street level to prevent flooding.
Several of the above projects have been implemented in numerous cities around the world, including many cities in Italy. The list of possible technical options is actually longer. For water resources management, one may mention the increase of the interconnectedness of water distribution networks, the minimization of leakage in water supply systems, the use of artificial water reservoir, the adaptation of agricultural techniques, precise irrigation and so forth.
Climate change adaptation is an example of no regret solution, namely, actions by households, communities, and local/national/international institutions that can be justified from economic, and social, and environmental perspectives whether natural hazard events or climate change (or other hazards) take place or not.
Climate change adaptation is an evolving field, which is relevant to society no matter if climate change is human induced or not. Adapting to the variability of climate has always been a target for humanity. The relevant research innovation that humans are continuously gaining opens new doors towards adaptation and therefore sustainability. The role of engineering in climate change adaptation is extremely important.
Design of adaptation strategies is a key step for reducing the impact of climate variability and change. Like any other process of engineering design, climate change adaptation requires the estimation of proper design variables alongside with their uncertainty. There is a fundamental difference - that is unfortunately often not properly considered - between engineering design and assessment of future climate sensitivity to human impact and other forcings. As it was mentioned in Section 6, the latter starts from assumptions that are not necessarily met in practice to produce what-if scenarios. Conversely, engineering design must be based on assessing and predicting the status of a given system the design refers to. Design variables are meant to summarise the above system status.
For the above reasons, climate models - designed to produce what-if scenarios - cannot be used alone for producing design variables as they are not conceived for simulating in detail the status of the system under design.
To identify technical methods that should be followed to reach the target of climate adaptation one needs to refer to the environmental and civil engineering literature and adapt the related design procedures to changing system behaviors. For most of the practical problems a simple adaptation of usual procedures, perhaps by introducing a proper safety factor - or allowance for freeboard - estimated on the basis of expert knowledge, may suffice.
Design of infrastructures in the presence of climate variability is frequently carried out in water resources management, by relying on stochastic simulation . The latter techinque is based on the assumption that the considered design variable is a random variable. This association is particularly useful in the presence of uncertainty. In fact, randomness is directly associated to the uncertain value of the considered variable. Stochastic simulation is a technique to trace the uncertain evolution of variables that can change stochastically (randomly) with certain probabilities.
The rationale behind stochastic simulation is to characterise the actual dynamics of relevant systems through an inductive approach, namely, through data driven techniques. Recent developments (Montanari and Koutsoyiannis, 2012) elaborated a framework that allows one to take into account physically based knowledge in inductive reasoning. That is, the available knowledge of the system is used to condition stochastic assessment and simulation.
Stochastic simulation is applied in classical water resources management by creating several future scenarios that are obtained by simulating observations that have not been observed, but are equally likely with respect to observed values. Namely, the simulated time series have the same statistics of historical data. These steps are repeated until a sufficient amount of data is gathered. The distribution of the outputs shows the most probable estimates as well as a frame of expectations regarding what ranges of values the variables are more or less likely to fall in.
In the presence of climate change, past and current system conditions may not be representative of what will occur in the future. However, they are still representative of system's behaviors and therefore their assessment is an essential step for deciphering how the system itself may react to change. Therefore, a possible opportunity for estimating design variables under changing condition may be articulated into three main steps which may be identified with the term "stochastic change simulation":
- Assessment of current system's conditions through stochastic analysis;
- Estimation of the impact of change on system's relevant statistics;
- Generation of future scenarios under change through stochastic simulation.
The first step above is carried out by using classical statistical analysis. The second step may be resolved by expert knowledge and/or assessing the output of climate models in statistical terms. A key requirement is uncertainty assessment for the above estimates of the impact of change. The third step can be again carried out through the usual stochastic simulation techniques.
Stochastic change simulation has the advantage of starting from a known situation, which is resembled by the data, rather than starting from assumptions and models whose uncertainty might be difficult to quantify. Also, stochastic change simulation has the advantage that feedbacks and process dynamics are intrinsically considered, as they are resembled by past history and our physical understanding that is incorporated into the stochastic model.
Stochastic change simulation is frequently employed in climate impact studies for generating weather variables. In most of the cases, future climate statistics are estimated by running climate models.
An interesting approach is stochastic simulation, which combines statistical and physically based modelling. Accordingly, the projections of future climate are obtained through a statistical approach which is obtained by projecting into the future the statistics of the past climate. These statistics can be corrected by taking into account the predictions of climate models when appropriate. An interesting example of this technique was recently provided by Kiem et al. (2021). From their abstract one reads:
" In this study, we develop an approach for stochastically generating future seasonal (monthly to annual) hydroclimatic conditions at multiple sites for water supply security assessment that capitalizes on an Australia‐wide relationship between annual average daily maximum temperature and annual rain (and flow). This approach is practical as it (i) avoids the extra time and additional uncertainties introduced by downscaling and bias correction of climate model produced rainfall information and (ii) takes advantage of the fact that climate model projections for temperature change are more realistic than climate model projections for rainfall."
Design of climate change adaptation strategies is a research field that is continuously evolving that opens the doors to exciting perspectives for the near future. In consideration of the on going developments, this lecture is continuously evolving as well. Date of last change is shown at the bottom of the page.
Arnell, N. W., & Gosling, S. N. (2013). The impacts of climate change on river flow regimes at the global scale. Journal of Hydrology, 486, 351-364.
Blöschl, G., & Montanari, A. (2010). Climate change impacts—throwing the dice?. Hydrological Processes: An International Journal, 24(3), 374-381.
Blöschl, G., Hall, J., Parajka, J., Perdigão, R. A., Merz, B., Arheimer, B., ... & Čanjevac, I. (2017). Changing climate shifts timing of European floods. Science, 357(6351), 588-590
Blöschl, G., Hall, J., Viglione, A., Perdigão, R. A., Parajka, J., Merz, B., ... & Boháč, M. (2019). Changing climate both increases and decreases European river floods. Nature, 573(7772), 108-111.
Brown, C., Ghile, Y., Laverty, M., & Li, K. (2012). Decision scaling: Linking bottom‐up vulnerability analysis with climate projections in the water sector. Water Resources Research, 48(9).
Chambers, DP, MA Merrifield, SR Nerem (2012) Is there a 60-year oscillation in global mean sea level? Geophysical Research Letters, 39, L18607.
Curry, J. (2018), Sea level and climate change, available online at https://curryja.files.wordpress.com/2018/11/special-report-sea-level-rise3.pdf.
Fasullo, JT, C Boening, FW Landerer, RS Nerem (2013) Australia’s unique influence on logal sea level in 2010-2011. Geophys. Res. Lett, 40, 4368-4373.
Guerreiro, S. B., Fowler, H. J., Barbero, R., Westra, S., Lenderink, G., Blenkinsop, S., ... & Li, X. F. (2018). Detection of continental-scale intensification of hourly rainfall extremes. Nature Climate Change, 8(9), 803.
Joseph, J., Ghosh, S., Pathak, A., & Sahai, A. K. (2018). Hydrologic impacts of climate change: Comparisons between hydrological parameter uncertainty and climate model uncertainty. Journal of Hydrology, 566, 1-22.
Horton, BP, RE Kopp, AJ Garner, et al. (2018) Mapping sea-level change in time, space and probability. Ann. Rev. Environ. Resourc., 43, 481-521.
Koutsoyiannis, D., Efstratiadis, A., Mamassis, N., & Christofides, A. (2008). On the credibility of climate predictions. Hydrological Sciences Journal, 53(4), 671-684.
Karger, D. N. et al. Climatologies at high resolution for the earth’s land surface areas. Sci. Data 4:170122 doi: 10.1038/sdata.2017.122 (2017).
Kiem, A. S., Kuczera, G., Kozarovski, P., Zhang, L., & Willgoose, G. (2021). Stochastic generation of future hydroclimate using temperature as a climate change covariate. Water Resources Research, e2020WR027331.
Lamb, H. H. (2002). Climate, history and the modern world. Routledge.
Montanari, A. (2012). Hydrology of the Po River: looking for changing patterns in river discharge. Hydrology and Earth System Sciences, 16(10), 3739-3747.
Montanari, A., & Koutsoyiannis, D. (2012). A blueprint for process‐based modeling of uncertain hydrological systems. Water Resources Research, 48(9).
Moon, H., Gudmundsson, L., & Seneviratne, S. I. ( 2018). Drought persistence errors in global climate models. Journal of Geophysical Research: Atmospheres, 123, 3483– 3496. https://doi.org/10.1002/2017JD027577.
Munk, W (2007) Sea level rise: an enigma. Proc. Nat. Acad. Sci., 99, 6550-6555.
Nijssen, B., O'donnell, G. M., Hamlet, A. F., & Lettenmaier, D. P. (2001). Hydrologic sensitivity of global rivers to climate change. Climatic change, 50(1-2), 143-175.
Padrón, R. S., Gudmundsson, L., & Seneviratne, S. I. ( 2019). Observational constraints reduce likelihood of extreme changes in multidecadal land water availability. Geophysical Research Letters, 46, 736– 744. https://doi.org/10.1029/2018GL080521.
Papalexiou, S. M., & Montanari, A. (2019). Global and regional increase of precipitation extremes under global warming. Water Resources Research, 55(6), 4901-4914.
Roderick, M. L., & Farquhar, G. D. (2002). The cause of decreased pan evaporation over the past 50 years. science, 298(5597), 1410-1411.
Scheraga, J. D., & Grambsch, A. E. (1998). Risks, opportunities, and adaptation to climate change. Climate research, 11(1), 85-95.
Stainforth, D. A., M. R.Allen, D.Frame, J.Kettleborough, C.Christensen, T.Aina, and M.Collins (2004), Climateprediction.net: A global community for research in climate physics, in Environmental Online Communication, edited by A.Scharl, pp. 101–112, Springer, London, U.K.
StainforthD. A., et al. (2007), Issues in the interpretation of climate model ensembles to inform decisions, Philos. Trans. R. Soc. A, 365, 2163–2177.
Vecchi, G. A., & Wittenberg, A. T. (2010). El Niño and our future climate: Where do we stand?. Wiley Interdisciplinary Reviews: Climate Change, 1(2), 260-270.
Veizer, J., Ala, D., Azmy, K., Bruckschen, P., Buhl, D., Bruhn, F., ... & Jasper, T. (1999). 87Sr/86Sr, δ13C and δ18O evolution of Phanerozoic seawater. Chemical geology, 161(1-3), 59-88.
Download the powerpoint presentation of this lecture
Last modified on Feb 28, 2021
- 38951 views